ChatGPT was down a couple of days ago, and I thought “I should take the day off, because I can’t get ANY work done.” I remember thinking the same thing about the internet in the early 2000’s.
It’s scary how fast ChatGPT has gone from a fun app to a tool that I can’t work without, similar to the internet. But I’m not scared of ChatGPT, because it’s not true artificial intelligence. It’s a word prediction tool…like the predictive text feature on my iPhone…but WAY more powerful.
As a daily Hacker News reader, I felt well read on AI and large language models (LLMs). But I didn’t grok how LLMs worked until I attended this year’s Texas Data Day. Here’s what I learned.
ELI5 (Explain Like I’m 5) Metaphor for LLMs:
Imagine you have a room filled with different kinds of toys: cars, dolls, balls, and blocks. Each type of toy can be sorted and grouped based on its features, like shape, color, or size. Think of your room as a big space where each area is reserved for a type of toy based on these features.
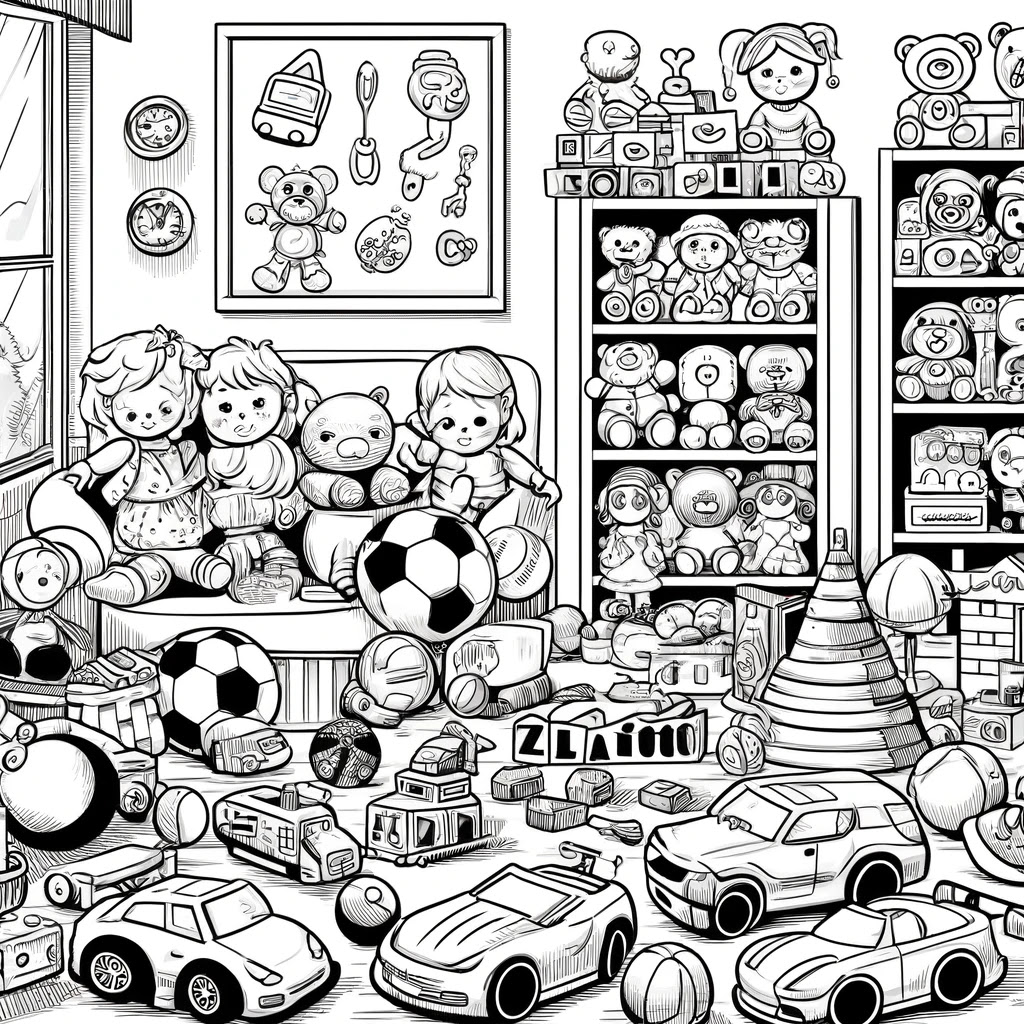
Turning Data into Vectors:
Because computers are good with numbers, we turn data, like toy characteristics, into numbers. For example, a car might be [1, 4, 7] based on its type, color, and size.

The Space (aka Your Room):
These numbers place toys in a “vector space,” where similar toys are closer. So, cars and trucks, both with wheels, might be near each other.

Training the LLM:
Computer scientists teach the LLM by showing it MANY examples of rooms, toys and their features. Then the model recognizes patterns, like toys with wheels, are similar.

Why It’s Useful:
When done, LLMs can do cool things like understanding and grouping new toys it hasn’t seen before. For instance, it recognizes that cars and trucks are similar because of their wheels, aiding in tasks like organizing toys or suggesting new ones similar to those you like.
It’s probably not a surprise, but I used ChatGPT to write the above metaphor and produce the images.
So What About Hallucinations?
Hallucinations are when LLMs return words or images that don’t make sense. Zooming into the images above, you’ll see good examples – things that kind of look like toys but not really.

Hallucinations are no reason to not use LLMs. They just mean that a human needs to apply a common sense check of the output…just like you’d do with any other tool.
So let’s go back to ChatGPT as a tool that I can’t work without. I mean, I could, but I don’t want to. Because working with ChatGPT is fun. It’s fun when it hallucinates, and I can feel superior in my highly evolved human intellect. It’s fun when it gives me tone-deaf advice, and I can tell it to f*** off and no one’s feelings get hurt. It’s fun when it catches my grammatical errors and keeps me from feeling dumb. And it’s really fun when ChatGPT saves me hours of work, and I feel like a superhuman at the end of the day.
If you haven’t incorporated ChatGPT, Gemini or other LLM into your daily workflow, here’s how to get started:
1. Open https://chat.openai.com/ in a new tab
- ChatGPT version 3.5 is free, but you SHOULD pay $20 for ChatGPT4 – and I don’t say that lightly as I understand the pain of having yet 1 more monthly subscription.
2. Keep ChatGPT open whenever you work or play online.
- In addition to googling for answers, ask your question of ChatGPT. And let me know if you run into anything scary.
If you have adopted an LLM into your daily workflow, send me your favorite tasks to complete more efficiently. I’m putting together a list to share as this one is long enough as is.